
Artificial Intelligence (AI) is rapidly becoming a cornerstone of innovation for healthcare providers of all sizes. For mid-sized hospitals and clinics, AI offers a chance to enhance efficiency, improve patient outcomes, and stay competitive without the massive budgets of large health systems. In 2025, this is more crucial than ever: AI technologies are already helping doctors spot fractures, triage patients, and detect early signs of disease (5 ways AI is transforming healthcare | World Economic Forum). Meanwhile, healthcare faces rising pressures – from a projected shortage of 10 million health workers by 2030 to higher patient expectations for convenient, personalized care. Mid-sized healthcare providers must leverage AI to bridge these gaps, streamline operations, and deliver high-quality care at lower cost. Below, we explore 5 AI solutions that are transforming mid-sized healthcare organizations today.
5 AI Solutions Transforming Mid-Sized Healthcare
1. AI-Powered Administrative Automation

One of AI’s most immediate impacts is in automating repetitive administrative tasks. Mid-sized providers often have limited staff juggling scheduling, billing, and record-keeping. AI-powered automation can take over many of these duties, reducing errors and freeing up staff for more valuable work. Key administrative areas being transformed by AI include:
Appointment Scheduling: Intelligent scheduling systems use AI to manage calendars and appointments. They can match patient requests with provider availability, send automated reminders, and even predict no-shows to overbook slots if needed. This not only saves front-desk staff time but also minimizes empty slots and waiting room bottlenecks. For example, AI-driven scheduling has been shown to reduce the effort of building staff schedules by 28% while ensuring staffing better meets patient needs.
Billing and Claims Processing: AI is streamlining the revenue cycle for mid-sized providers. AI-driven billing systems automatically capture charges, verify insurance details, and submit claims with minimal human input. They can flag errors in real-time (such as missing patient info or coding mistakes) to prevent denials. By handling claims and reimbursements faster and more accurately, these systems reduce administrative overhead. Notably, AI-powered claims processing cuts down on manual work and lowers the risk of denied claims due to billing errors. This means quicker payments and improved cash flow – a big win for resource-strapped clinics.
Documentation and Record Management: Healthcare is infamous for its paperwork. AI tools using natural language processing (NLP) can transcribe and summarize clinical notes, or auto-fill forms based on prior data. Other AI systems digitize and organize patient records, making data easier to search and analyze. By automating data entry and document processing, AI ensures records are up-to-date and accessible. This improves compliance and saves countless hours of clerical work. Crucially, by taking on tedious documentation tasks, AI allows nurses and administrators to focus more on patient care rather than paperwork.
For mid-sized healthcare organizations, automating administrative workflows isn’t just about convenience – it’s become a strategic necessity. These providers operate with tighter margins and smaller staffs than large hospital systems. AI-powered administrative automation boosts operational efficiency and accuracy, which in turn improves patient satisfaction (fewer billing errors, shorter wait times) and staff morale. Importantly, it levels the playing field: small and mid-sized providers can achieve back-office efficiency closer to that of larger institutions. In 2025’s challenging healthcare climate, every saved dollar and hour counts, and AI is helping unlock those savings in the administrative realm.
2. Predictive Analytics for Patient Care

Another game-changing application of AI in healthcare is predictive analytics – using machine learning to analyze historical and real-time data to forecast future events. For mid-sized providers, predictive analytics can be a powerful tool to improve patient care and optimize operations. AI-driven predictive models scour through electronic health records, demographics, and even social data to find patterns that humans might miss. The result is actionable insights that help clinicians and managers make proactive decisions. Major uses of predictive analytics include:
Early Disease Detection: By analyzing vast datasets (medical histories, genetic information, population health trends), AI can identify subtle indicators that a patient may be developing a condition long before clinical symptoms manifest. For example, researchers have developed AI models that can detect signatures of over 1,000 diseases years in advance of symptoms, predicting with high confidence a future diagnosis. This kind of early warning allows for truly preventive care – clinicians can monitor at-risk patients more closely or start interventions sooner, potentially halting disease progression. In practice, this could mean catching cancers at Stage 1 instead of Stage 3, or identifying early signs of chronic illnesses (like diabetes or heart failure) so that lifestyle changes or medications can be introduced in time to make a difference. Early detection through AI not only saves lives but also reduces long-term treatment costs.
Preventing Hospital Readmissions: Predictive analytics is being used to forecast which patients are at highest risk of complications or readmission after discharge. Hospitals can then target those patients with extra support – follow-up calls, home health visits, tailored care plans – to prevent avoidable readmissions. This is especially valuable for mid-sized hospitals facing penalties for high readmission rates. In one real-world example, Corewell Health (a regional health system) applied AI-powered risk scoring to discharged patients and managed to keep 200 high-risk patients from being readmitted, resulting in $5 million in cost savings. By working from day one of discharge to address recovery barriers, the care team was able to significantly reduce actual readmissions among those flagged as high-risk. This case illustrates how predictive analytics can improve outcomes (patients staying healthier at home) while also protecting the hospital’s bottom line.
Resource Allocation & Operational Forecasting: Beyond direct patient care, predictive models help mid-sized providers optimize their use of resources. AI can analyze patterns in admissions, seasonal illness surges, and local demographics to forecast patient volumes. This enables hospitals to adjust staffing and inventory in advance. For instance, predictive analytics might show that emergency department visits tend to spike on certain days or that flu cases will rise 3 weeks from now – allowing management to schedule additional staff or stock extra supplies accordingly. AI is also used to predict operating room demand, bed occupancy, and even equipment maintenance needs. By anticipating what will be needed, mid-sized facilities can allocate nurses, doctors, and equipment more efficiently, reducing wait times and avoiding resource shortages. In essence, these analytics turn data into a crystal ball, guiding hospitals to be prepared instead of reactive. This leads to smoother operations and better patient service despite the unpredictable nature of healthcare.
In summary, predictive analytics empowers mid-sized healthcare providers to move from a reactive stance to a proactive one. Rather than waiting for a patient to crash or an ER to overflow, AI-driven predictions give an early heads-up, whether it’s a patient likely to develop complications or a trend toward increased service demand. By acting on these forecasts – intervening early or reallocating resources – providers can improve patient outcomes (fewer emergencies and complications) and use their limited resources more wisely. As data becomes more abundant and AI models grow more sophisticated, predictive analytics will increasingly guide everyday decisions in mid-sized healthcare, making care more anticipatory and personalized.
3. AI-Driven Diagnostics & Clinical Decision Support
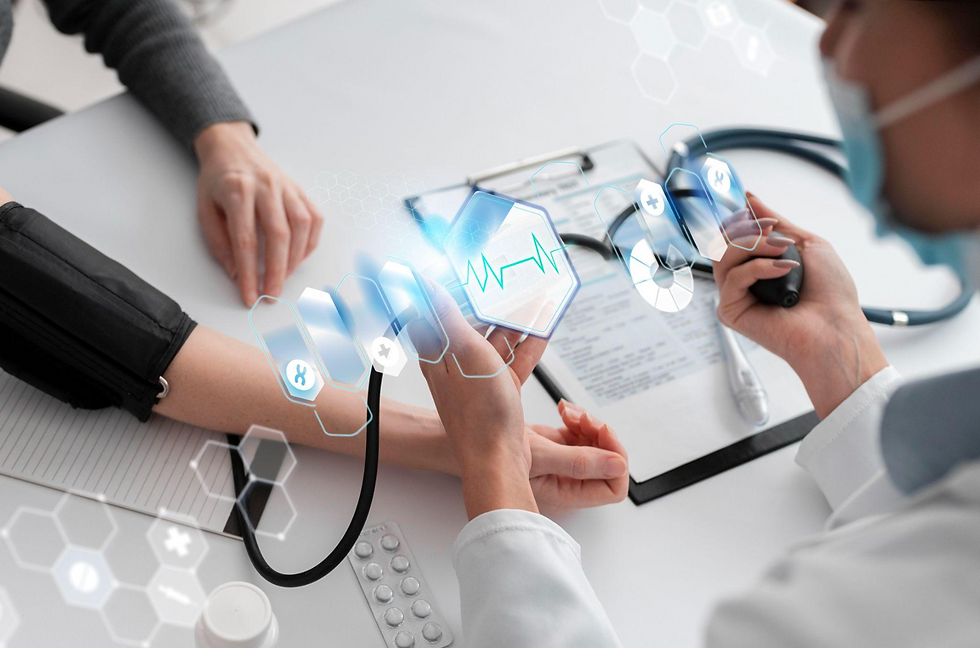
AI is revolutionizing how clinicians diagnose diseases and make treatment decisions. Mid-sized hospitals may not always have a full roster of sub-specialist physicians on hand, but AI tools can act as force multipliers – offering expert-level insights in medical imaging, risk assessment, and evidence-based recommendations at the point of care. Two of the most impactful areas in this domain are AI-assisted diagnostics (especially in medical imaging) and AI-enhanced clinical decision support systems (CDSS).
AI in Medical Imaging and Diagnosis: Machine learning algorithms excel at pattern recognition, which makes them incredibly useful for analyzing medical images like X-rays, CT scans, MRIs, and pathology slides. AI-powered image analysis can detect abnormalities with speed and precision, sometimes catching details that escape the human eye. In fact, studies have shown that AI is now competitive with, or even superior to, human experts in certain diagnostic tasks – for example, algorithms have outperformed doctors in identifying malignant tumors in imaging data. A recent case in the UK highlighted AI’s potential: an AI software was “twice as accurate” as professionals at examining brain scans of stroke patients. It not only identified strokes more accurately, but also determined the timing of the stroke, a critical factor for deciding treatments. In another instance, AI tools are helping to spot fractures on X-rays that busy emergency clinicians might miss. Urgent care doctors miss up to 10% of fractures on initial reading, but using AI for the first review can avoid those missed injuries; the UK’s National Institute for Health and Care Excellence (NICE) found such AI tech to be safe, reliable, and able to reduce unnecessary follow-ups from misread X-rays. For mid-sized facilities, implementing these AI diagnostic aids means patients get faster, more accurate answers. An AI can flag a suspicious lung nodule on a chest X-ray or an early stroke on a CT scan within seconds, prompting the radiologist or neurologist to act immediately. It’s like having an extra pair of expert eyes on every image, 24/7. This not only improves diagnostic accuracy but also helps address staff shortages – one AI system can effectively support many clinicians, ensuring nothing is overlooked even during off-hours.
AI in Clinical Decision Support: Beyond images, AI is augmenting clinical decision-making through advanced decision support tools. These AI-driven CDSS analyze patient data (symptoms, vitals, lab results, medical history) and cross-reference it with vast medical knowledge bases and prior outcomes to assist providers in choosing optimal care paths. For example, an AI-based system can assess a hospitalized patient’s records and alert the care team that the patient’s risk of developing sepsis is high – before the patient actually shows severe symptoms – enabling preventive measures. Similarly, AI can help stratify patient risk (like predicting which ICU patient is likely to deteriorate in the next 12 hours) so that clinicians can prioritize attention appropriately. Some decision support AIs can suggest potential diagnoses a doctor might not have considered, or recommend treatment adjustments based on predictive models (e.g., warning that a certain patient is unlikely to respond to a standard medication). Importantly, these tools don’t replace the physician’s judgment; rather, they provide an evidence-backed second opinion in real time. They draw on data from thousands of cases, medical journals, and clinical guidelines in seconds – something no individual human could do in a moment of decision. By integrating with electronic health records, AI decision support can present these insights during the patient encounter, such as a pop-up that alerts a doctor to a possible drug interaction or a reminder about a care guideline for a patient’s condition. For mid-sized providers, which may not have specialists for every niche scenario, this leveled-up decision support ensures patients still receive care informed by the latest knowledge and analytics. It reduces diagnostic errors and variance in care quality by providing consistent, data-driven suggestions. Ultimately, AI in diagnostics and decision support acts as a safety net and an accelerant: catching what humans might miss, and speeding up the recognition-to-treatment cycle. Patients benefit through more accurate diagnoses, earlier interventions, and personalized treatment plans – no matter if they’re at a small community hospital or a large academic center. As these AI tools become more widespread, mid-sized healthcare providers can deliver cutting-edge diagnostic care without needing on-site specialty experts for every condition.
4. AI for Telehealth & Remote Patient Monitoring
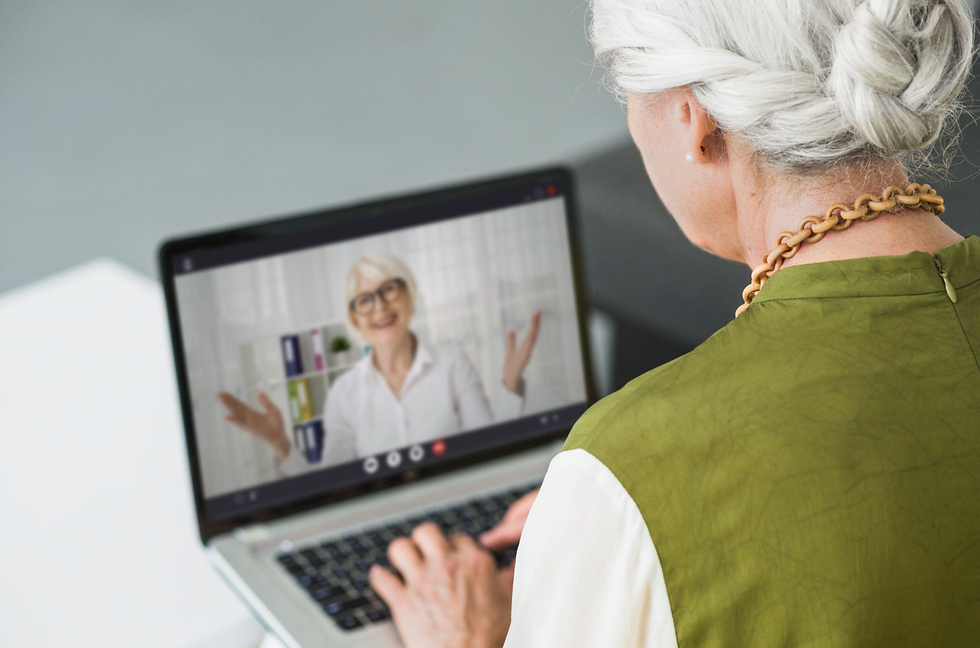
Telehealth exploded in usage during the COVID-19 pandemic and has since become a mainstay of healthcare delivery. Mid-sized healthcare organizations have embraced virtual care to extend their reach and offer patients convenience. Now, AI is taking telehealth and remote patient monitoring (RPM) to the next level by improving patient engagement, triage, and continuous care beyond the hospital walls. From intelligent chatbots that converse with patients to wearable sensors that alert clinicians in real time, AI is making “care anywhere” more effective and scalable.
Virtual Triage Assistants and Chatbots: One way AI is enhancing telehealth is through chatbot “virtual assistants” that interact with patients via text or voice. These AI chatbots can ask patients about their symptoms, answer common health questions, and provide guidance on whether a clinic visit is needed – all available 24/7. This around-the-clock availability means patients can get immediate answers at any time, even when offices are closed, without tying up staff. For mid-sized providers, an AI chatbot can handle a large volume of routine inquiries in a standardized way, which reduces the call burden on nurses and front-desk staff. Patients benefit by not having to wait on hold or until morning for minor concerns. For instance, if a patient texts a clinic at 9 PM about a rash or a fever, the AI assistant can run through triage questions and either provide home care advice, schedule an appointment, or direct the patient to urgent care if needed. These systems use vast medical databases to provide reliable information and can escalate to human providers if they detect a serious issue. Studies show that when implemented properly, chatbots can resolve many patient queries without a live agent, improving efficiency. Moreover, chatbots can help with administrative tasks like appointment booking via simple back-and-forth messaging, or sending medication reminders and follow-up prompts. This kind of virtual navigation eases the patient’s journey through the healthcare system and keeps them engaged in their care.
Remote Patient Monitoring with AI: For patients with chronic conditions or those recovering at home, remote patient monitoring has emerged as a vital service. Patients use connected devices – like wearable heart monitors, blood pressure cuffs, glucose sensors, or even smartphone apps – that continuously or regularly record health metrics and send them to providers. AI comes into play by analyzing this steady stream of data in real time to detect worrisome changes. Instead of a nurse manually checking dozens of readings, an AI system can instantly flag that an elderly patient’s blood pressure has been trending upward for days or that a patient with COPD’s oxygen levels have dipped below a threshold. This allows care teams to intervene early, often preventing an acute exacerbation or hospital readmission. In practical terms, if a remote monitor reports subtle signs of heart failure worsening, the AI could prompt a nurse to call the patient to adjust medications or bring them in before they land in the ER. The impact on outcomes is significant: A study of Mayo Clinic’s COVID-19 RPM program found that high-risk patients who actively engaged with AI-supported remote monitoring had significantly lower rates of hospitalization and even mortality compared to those who did not engage. Essentially, the technology enabled staff to catch deteriorations sooner and alter the disease trajectory with timely interventions. Even outside of pandemic use, similar programs for chronic diseases have shown reductions in ER visits and hospital days. For mid-sized healthcare providers, RPM augmented by AI is a force multiplier – a single centralized care team can effectively oversee hundreds of patients at home, with AI prioritizing which patients need attention first based on the data. This means better care for patients (who feel looked after between visits and can avoid complications) and more efficient use of clinical staff (who focus only on patients who need action). It also expands a provider’s capacity – instead of being limited by beds or exam rooms, they can monitor many more patients remotely.
Enhanced Patient Engagement and Access: AI in telehealth also boosts patient engagement. Personalized health coaching chatbots, for example, can check in with patients regularly to encourage medication adherence, diet and exercise, or mental health check-ins. These AI-driven interactions keep patients involved in their care plan and can alert clinicians if a patient reports concerning symptoms or non-compliance. Additionally, AI translation tools can enable telehealth services across multiple languages, helping mid-sized providers serve diverse communities without needing an on-site interpreter for every call. By lowering communication barriers and automating follow-ups, AI ensures more patients stay connected to their providers. Ultimately, AI-driven telehealth and RPM allow mid-sized healthcare organizations to offer “hospital-grade” oversight and interactive care to patients at home or on the go. Geography becomes less of a limitation – a rural clinic can use AI triage to serve remote patients, and a specialty center can remotely manage a patient’s condition in between in-person visits. This hybrid model of care, powered by AI, leads to earlier interventions, more convenient service, and a patient experience that is both high-tech and highly personalized.
5. AI in Cybersecurity & Compliance
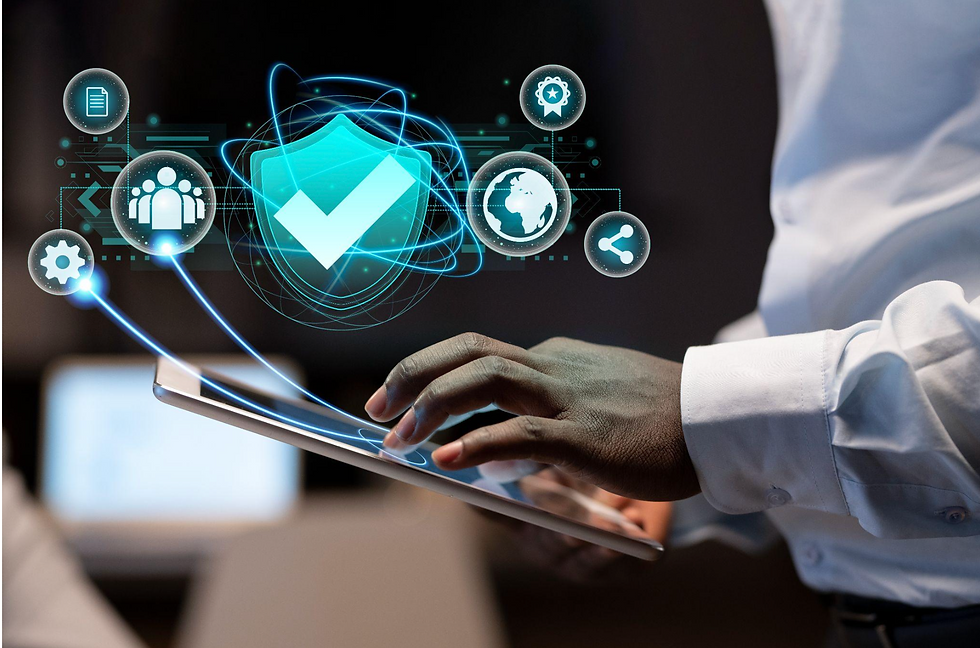
As healthcare becomes more digital, mid-sized providers face growing challenges in protecting sensitive patient data and meeting strict regulatory requirements (like HIPAA). Cyberattacks on healthcare – from ransomware shutting down hospital systems to hackers stealing patient records – have surged in recent years. The financial and reputational damage from a breach can be devastating: healthcare data breaches are the costliest of any industry, with the average breach in 2023 costing about $10.93 million. Mid-sized organizations, which may lack the extensive IT security teams of larger systems, are especially vulnerable. AI offers advanced solutions to bolster cybersecurity defenses and ensure compliance is maintained automatically.
AI-Powered Threat Detection and Response: Traditional security tools rely on known threat signatures and static rules, which often fail to catch novel or sophisticated attacks. AI changes the game by using machine learning to monitor network traffic, user behavior, and system logs in real-time, identifying anomalies that could signal a cyber intrusion. These AI systems “learn” what normal activity looks like in a hospital’s IT environment – such as typical log-in times, patterns of data access, and network usage. When something deviates from the norm (say, an employee account suddenly downloading a huge volume of records at 2 AM, or an unfamiliar program trying to modify files), the AI flags it or takes action. This continuous monitoring and pattern-recognition capability means threats can be detected within moments of their onset. In many cases, AI can surface subtle indicators of an attack that a human admin might overlook until it’s too late. For example, a major French hospital group recently thwarted a ransomware attack in its early stages thanks to an AI cybersecurity system that immediately detected unusual file activity before the attack spread. That kind of rapid detection and response can literally prevent a disaster – avoiding downtime of clinical systems or the exposure of thousands of patient records. AI security platforms can also automatically isolate infected devices or block suspicious network connections the instant a threat is sensed, containing damage while alerting IT staff. For mid-sized providers, deploying AI for threat detection is like having a virtual security analyst on duty 24/7, tirelessly guarding the gates. This is crucial when budget or personnel constraints mean you can’t have a large round-the-clock security operations center. AI essentially augments a lean IT team, ensuring that any breach attempt is noticed and stopped in its tracks if possible.
Compliance Monitoring and Data Protection: Compliance with healthcare regulations (HIPAA, GDPR for those serving international patients, etc.) is non-negotiable, but the requirements can be arduous. AI helps automate compliance by tracking and controlling access to sensitive data and maintaining detailed logs. AI-driven systems can implement role-based access control – ensuring staff members only access the minimum necessary information for their role – and instantly flag if someone attempts to access records outside their privilege level. They also create comprehensive audit trails by recording every access, modification, or transfer of patient data in real-time. This makes it vastly easier to generate compliance reports or investigate a potential privacy incident, as the AI has documented who did what and when in a centralized log. Additionally, AI aids in data encryption and privacy. It can automatically encrypt data at rest and in transit, applying the latest encryption standards consistently across systems. In the event of a breach, encrypted data is useless to thieves, thereby protecting patient confidentiality. AI algorithms can even scan outgoing emails or file transfers to detect unintentional inclusion of patient identifiers, preventing accidental HIPAA violations (for instance, warning if a doctor is about to email an unencrypted spreadsheet with patient info). Another aspect is behavioral analytics for insider threats – AI monitors user behavior within systems and spots anomalies that might indicate an insider misuse or a hacked account, such as a clerk attempting to download an entire patient database. This proactive approach catches internal compliance issues that might otherwise fly under the radar.
For mid-sized healthcare organizations, which often operate with a small IT/security team, AI-driven cybersecurity and compliance tools are invaluable guardians. They reduce the likelihood of costly breaches by acting as an ever-vigilant watchdog that can react in milliseconds. They also ease the burden of compliance by automating many security policies and record-keeping requirements, ensuring nothing falls through the cracks. By investing in AI for cybersecurity, mid-sized providers protect their patients’ trust – and avoid devastating financial hits – while keeping their operations running smoothly. In an era where cyber threats are growing in number and complexity, AI is quickly becoming the linchpin of a robust defense strategy in healthcare.
Case Studies & Real-World Applications
Mid-sized healthcare providers across the globe are already reaping tangible benefits from implementing these AI solutions. Here are a few real-world examples that showcase how AI is making a difference, along with the measurable impact:
Administrative Automation – Assuta Medical Centers: A mid-sized health system in Israel (eight facilities, ~100k procedures a year) partnered with an AI company to automate nurse scheduling. After rolling out the AI scheduling platform, Assuta’s managers reported a 28% reduction in time and effort spent building schedules, and a 14% increase in confidence that staffing levels matched patient care needs. The AI algorithm predicted unit-level workloads and optimized shift assignments accordingly, resulting in more equitable and efficient use of their nursing staff. This real-world deployment shows how even a mid-sized provider can leverage AI to solve chronic scheduling headaches, improving both productivity and staff satisfaction while reducing burnout.
Predictive Analytics – Corewell Health’s Readmission Reduction: Corewell Health (formed from Spectrum Health, a regional system) used AI-driven predictive analytics to tackle hospital readmissions. In 2021, their care management team started using an AI model to identify recently discharged patients at high risk of readmission and provided targeted support to those patients. The results were striking: over 200 patients were prevented from being readmitted, saving an estimated $5 million in costs. By forecasting which patients would struggle after discharge – using factors like clinical condition, social determinants, and prior utilization – the health system intervened early (e.g., arranging follow-ups, home care, transportation for appointments). This case study demonstrates that mid-sized and regional providers can deploy predictive AI to drive down readmissions, improving patient outcomes and avoiding penalties or losses from uncompensated readmissions.
AI-Driven Diagnostics – Stroke Detection Trial: In a collaboration between two UK university hospitals, an AI software was tested as a diagnostic aid for stroke patients. Trained on hundreds of brain scans, the AI was used on 2,000 real patient scans and proved to be twice as accurate as human specialists in identifying strokes on CT images. Moreover, it could determine the timing of the stroke’s occurrence, which is critical for treatment decisions. This trial led to growing confidence in AI’s diagnostic abilities and paves the way for hospital adoption of such tools. It’s a compelling example of how even mid-sized hospitals (which these university hospitals were in terms of regional size) can incorporate AI to augment their diagnostic capabilities. With stroke being a time-sensitive diagnosis, having an AI effectively act as a second expert radiologist can significantly speed up treatment for better patient outcomes.
Telehealth & Remote Monitoring – Mayo Clinic’s RPM Program: Mayo Clinic (while a large academic center, its RPM program offers a model that mid-sized systems have also emulated) implemented an AI-enhanced Remote Patient Monitoring program during COVID-19 for patients recovering at home. Among nearly 5,800 high-risk COVID patients enrolled, those who actively engaged with the remote monitoring (reporting symptoms and vitals via AI-guided apps and devices) had a significantly lower 30-day mortality rate (0.5% vs 1.7%) and were far less likely to be hospitalized or need ICU care than patients who did not engage. This real-world data underscores how AI plus telehealth can improve outcomes. Closer to the mid-sized scale, many community hospitals have since launched similar AI-backed monitoring for chronic disease management – for example, monitoring heart failure patients’ weights and blood pressures at home to preempt flare-ups. These programs have shown reductions in ER visits and shorter hospital stays for chronic disease patients. It proves that virtual care with AI oversight isn’t just a nice-to-have; it directly translates to lives saved and complications avoided.
Cybersecurity & Compliance – Ransomware Prevention at a Hospital: A notable success story in cybersecurity comes from a French hospital group that faced an attempted ransomware attack. The hospital had an AI-based security system (Darktrace) deployed, which immediately detected the early signs of the attack (suspicious files and behaviors) and alerted the IT team, who contained the threat before it could encrypt hospital data. In this case, AI potentially saved the hospital from a catastrophic shutdown. Closer to home, mid-sized U.S. hospital networks are increasingly using AI-driven security tools that have successfully caught malware and insider threats. For instance, one regional medical center reported that after implementing an AI monitoring system, it was able to identify an employee’s compromised account within minutes when it started downloading unusual amounts of data, allowing the breach to be stopped before any patient information was leaked. These examples highlight that AI is not theoretical in the security realm – it’s actively safeguarding healthcare facilities. Especially for mid-sized providers that might be targets for cybercriminals (viewed as “easier” than large systems but still rich in data), AI provides a much-needed defensive shield, as evidenced by these real-world interventions.
These case studies illustrate a common theme: mid-sized healthcare organizations can and do achieve significant improvements by adopting AI solutions. Whether it’s a scheduling system in a community hospital or a predictive model in an regional health network, the technology is yielding concrete results – faster operations, cost savings, better patient outcomes, and enhanced safety. They also show that AI is versatile across many aspects of healthcare. Each provider leveraged AI in a way that addressed a key pain point or strategic goal for their situation. The takeaway for mid-sized providers is that AI isn’t just hype; when implemented thoughtfully, it delivers measurable value. And as success stories accumulate, they provide roadmaps and confidence for other mid-sized organizations to follow suit with their own AI initiatives.
Future Implications: The Next 5 Years in Mid-Sized Healthcare
Looking ahead, the influence of AI on mid-sized healthcare is poised to deepen even further. The period through 2030 will likely be defined by broader AI adoption, more advanced AI capabilities, and closer integration of AI into every facet of healthcare delivery. Here are some key ways these AI solutions are expected to evolve and shape mid-sized healthcare in the next five years:
Mainstreaming of AI Across Operations: What is innovative today may be standard practice tomorrow. We’re already seeing a surge in the use of AI for personalized medicine, predictive analytics for early disease detection, and AI-powered clinical decision support – trends that analysts expect to accelerate. In the near future, mid-sized providers won’t just pilot AI in one department; they will run multiple AI tools in production, from the back office to the bedside. As proof-of-concepts turn into fully deployed solutions, AI will become as routine as EHR systems in clinical workflows. This could mean, for example, that every care team meeting includes AI-generated risk reports on patients, or that every claims batch runs through an AI audit before submission. The cumulative effect will be healthcare environments that are smarter and more responsive. Providers who embrace this will likely see marked improvements in efficiency and patient outcomes, while those slower to adopt may find themselves lagging in quality or cost-effectiveness.
Advances in AI Technology – from Generative AI to Digital Twins: The AI tools of 2025 and beyond will be more powerful and more intuitive. Generative AI (the technology behind sophisticated chatbots like GPT) is set to play a big role in healthcare administration and documentation. In the coming years, we can expect generative AI to draft clinical notes, summarize patient visits, or even create first drafts of discharge instructions and compliance reports, all based on conversational inputs from clinicians. This will significantly lighten documentation burdens – doctors might spend minutes reviewing AI-prepared notes instead of hours writing them from scratch (with the AI continually learning to improve the more it’s used). Moreover, generative AI can aid in creating educational materials or simulating patient scenarios for training. Another cutting-edge concept on the horizon is the digital twin of healthcare operations – a virtual replica of a hospital’s systems that can be used to simulate changes and forecast outcomes. Within five years, mid-sized hospitals could utilize AI-powered digital twins to test operational tweaks (like a new clinic workflow or a different staffing model) in a risk-free virtual environment before applying them in real life. This would enable evidence-based decision-making at an unprecedented level, essentially letting administrators “try before they buy” any major process change. Additionally, AI-driven robotics and automation will grow: expect to see more AI-assisted surgical robots in operating rooms of even mid-sized hospitals, or autonomous systems delivering supplies and medications in hallways. As these advanced technologies become more affordable and user-friendly, mid-sized facilities can adopt them without needing massive R&D budgets.
Greater Integration and Interoperability: In the future, AI solutions will not live in silos but will be seamlessly integrated with existing health IT systems and with each other. We can anticipate that electronic health record vendors will bake more AI features directly into their platforms – some EHRs are already adding built-in predictive analytics and voice-recognition AI for note-taking. This means mid-sized providers won’t always need to seek external AI tools; the capability will be part of their standard software, making adoption easier. Additionally, different AI systems will talk to one another. For example, an AI that forecasts patient admissions could automatically inform the AI scheduler to adjust staffing, or an AI that flags a patient’s deteriorating condition could directly trigger an alert in the clinician’s decision support dashboard. Such interoperability will create a cohesive AI-driven ecosystem in hospitals where insights flow quickly to the point of action. Over the next five years, standards and protocols for health AI integration (likely supported by industry groups and regulators) will mature, smoothing out data sharing and reducing the technical burden on mid-sized IT departments. The result will be that mid-tier providers can plug-and-play certified AI modules with confidence that they will safely enhance – not disrupt – their workflows.
Focus on Ethics, Bias, and Regulation: As AI use expands, there will be a parallel emphasis on ensuring these tools are safe, fair, and transparent. Regulators in the late 2020s will likely issue clearer guidelines on AI in healthcare, possibly requiring validation of algorithms for bias and robustness. Mid-sized providers will benefit from this increased oversight, as it will become easier to trust that an AI product on the market meets certain standards. Many organizations will also develop AI governance committees to oversee AI deployments, addressing questions like: How do we explain an AI’s recommendation to a patient? How do we handle disagreements between AI advice and clinician judgment? How do we continuously monitor AI performance? In the next five years, expect AI to become a regular topic in healthcare quality and compliance meetings. By proactively addressing these concerns, mid-sized providers will be able to harness AI’s benefits while mitigating risks. On the flip side, AI itself will help with compliance – for example, future AI could automatically detect if its own predictions are veering off (perhaps due to data drift) and alert the team to retrain the model, thus maintaining safety.
Continued Improvement in Outcomes and Efficiency: Perhaps the most exciting implication is how patient care could transform. If we project current trends forward, five years from now a mid-sized hospital might routinely achieve metrics that are ambitious today – think 30-40% lower readmission rates for chronic disease patients due to AI-guided interventions, or near-zero waiting time for appointments thanks to AI-driven scheduling and virtual care options. Quality of care could become more consistent as AI decision support reduces variability between providers. Mid-sized facilities will also find new opportunities: AI could enable them to offer specialized services (like remote radiology consults or AI-guided rehab programs) that previously only big centers could provide, thereby expanding their service lines and patient reach. With AI handling much of the drudgery and number-crunching, human clinicians and staff can operate at “top of license,” focusing on empathy, complex decision-making, and innovation. This human-AI partnership stands to create a more sustainable work environment – hopefully reducing burnout – and a more personalized patient experience. Over the next five years, as these technologies mature, mid-sized providers that invest in AI will likely see substantial ROI in terms of cost savings, revenue growth (through improved throughput and new services), and patient loyalty, as outcomes and experiences improve.
In summary, the next half-decade will likely take AI in healthcare from a set of separate impressive tools to a cohesive, omnipresent assistant in the healthcare ecosystem. Mid-sized healthcare organizations have a lot to gain: they can be nimble adopters of proven innovations, leveraging cloud-based AI solutions without the legacy baggage of larger systems. The playing field in healthcare could level out to some extent, as AI allows smaller providers to punch above their weight in care quality and efficiency. However, success will require strategic planning, wise choice of technologies, and ongoing commitment to training and integrating these tools. The hospitals and clinics that navigate this well will be the ones setting benchmarks in 2030. In essence, AI will continue to evolve from helping with individual tasks to orchestrating broader improvements in how healthcare is delivered – and mid-sized providers are squarely in that transformation journey.
Conclusion & Call to Action
AI is no longer a futuristic buzzword – it’s here and now, actively transforming how mid-sized healthcare providers operate and care for patients. From automating back-office tasks to predicting clinical outcomes and strengthening data security, the five AI solutions discussed are driving better efficiency, better decisions, and better results in healthcare. Mid-sized organizations stand at a pivotal moment: those that embrace these AI-driven innovations position themselves to thrive in an increasingly complex and competitive healthcare landscape. They’ll enjoy streamlined processes, healthier patient populations, and more empowered staff. Those that hesitate may find it harder to meet the rising standards of care quality and operational excellence that AI-equipped peers will achieve.
If you’re part of a mid-sized healthcare organization, the time to explore AI opportunities is now. Start with the pain points or strategic goals that matter most – whether it’s reducing burnout in your team or improving patient retention – and consider how an AI solution might help. Small pilots in one department can demonstrate value and build confidence for broader adoption. As highlighted, you don’t have to do it alone: the industry is rich with case studies and proven tools, and expertise is available to guide implementation.
Follow Mandelbulb Technologies to stay updated with the latest insights, success stories, and practical tips on healthcare AI. We regularly share industry trends and how-tos that can spark ideas for your own organization’s AI journey. And when you’re ready to take the next step, don’t hesitate to book a consultation with our team. We specialize in helping mid-sized healthcare providers tailor AI solutions to their unique needs – whether it’s choosing the right technology, navigating integration with your systems, or training your staff to work alongside AI. By taking action today, you can secure a smarter, more efficient, and innovative future for your healthcare organization. The promise of AI in healthcare is tremendous, and with the right partnership and strategy, you can turn that promise into reality – improving care for your patients and driving success for your organization. Here’s to a future where mid-sized healthcare is transformed and thriving, powered by the best of human compassion and artificial intelligence working hand in hand.
References
World Health Organization (WHO). (2023). Digital Health and Telemedicine: Closing Gaps in Primary Care.https://www.who.int/digital-health-telemedicine
Cited for the rise of telehealth and its role in improving patient engagement and access.
Deloitte. (2022). Global Health Care Outlook: Accelerating AI Adoption.https://www2.deloitte.com/global/en/pages/life-sciences-and-healthcare.html
Referenced for data on AI solutions in healthcare and projections of ROI for mid-sized hospitals.
Sage Growth Partners. (2021). Study: AI in Healthcare Administration.https://www.sage-growth.com/ai-healthcare-administration-study
Supports claims on how AI-powered automation (RPA/chatbots) reduces billing errors and appointment no-shows.
American Hospital Association (AHA). (2023). Hospitals’ Use of Data and Technology.https://www.aha.org/hospitals-and-health-systems-data
Used for statistics on administrative overhead and operational waste in mid-sized healthcare facilities.
Institute of Medicine (IOM). (2019). Best Practices for AI in Clinical Decision Support.https://www.nationalacademies.org/iom/ai-clinical-decisions
Referenced for examples of AI’s impact on diagnostic accuracy and how decision support tools can reduce errors.
Becker’s Hospital Review. (2023). AI Trends: 5 Use Cases Transforming Healthcare This Year.https://www.beckershospitalreview.com/healthcare-ai
Provided examples of administrative automation and predictive analytics used by mid-size providers.
McKinsey & Company. (2022). The Future of Healthcare AI: Operational Efficiency and Clinical Impact.https://www.mckinsey.com/industries/healthcare-systems-and-services/our-insights
Cited for statistics on AI-driven cost savings, improved patient outcomes, and adoption challenges.
IBM Watson Health. (2020). AI Diagnostics: From Image Recognition to Full Clinical Support.https://www.ibm.com/watson-health/clinical-ai-diagnostics
Used to illustrate AI imaging breakthroughs and improvements in diagnostic accuracy rates for mid-sized healthcare.
Harvard Business Review (HBR). (2021). How AI Is Personalizing Healthcare: Telehealth & Beyond.https://hbr.org/healthcare-ai-telehealth
Discussed the expansion of telehealth and remote patient monitoring, showing examples of improved patient satisfaction.
Health Affairs. (2022). How Hospitals Are Using AI for Remote Patient Monitoring and Reduced ER Visits.https://www.healthaffairs.org/remote-monitoring-ai-studies
Provided data on reduced readmissions and ER visits tied to AI-enhanced RPM programs.
Ponemon Institute & IBM Security. (2023). Healthcare Data Breach Costs and Prevention: AI & Compliance Trends. https://www.ibm.com/security/data-breach/healthcare
Referenced for the average cost of healthcare data breaches and the role of AI in threat detection.
Cybersecurity & Infrastructure Security Agency (CISA). (2022). Cyber Threats in Healthcare. https://www.cisa.gov/healthcare-cyber-threats
Covered cybersecurity threats facing hospitals and how AI-driven threat detection systems reduce risks.
American Medical Informatics Association (AMIA). (2021). Leveraging AI for Regulatory Compliance in Healthcare. https://amia.org/publications/ai-reg-compliance
Provided examples of automated compliance checks and data management strategies using AI.
PwC Health Research Institute. (2023). Adopting AI in Mid-Sized Hospitals: Key Barriers and Enablers. https://www.pwc.com/us/en/health-industries/ai-mid-size-hospitals.html
Discussed the challenges of implementing AI solutions in smaller facilities with limited budgets or IT resources.
Accenture. (2022). Healthcare’s New Normal: AI for Clinical and Operational Gains. https://www.accenture.com/us-en/insights/health/ai-healthcare-new-normal
Provided data on how AI-based solutions are improving patient satisfaction and operational KPIs in hospitals.
Healthcare Information and Management Systems Society (HIMSS). (2023). Reducing Administrative Burden with AI & RPA. https://www.himss.org/resources/ai-rpa-healthcare-administrative-burden
Referenced for the estimate that up to 40% of hospital expenses are tied to administrative tasks and how AI can reduce that load.
Epic Research. (2021). Predictive Analytics for Readmission Reduction: A Multi-Hospital Study. https://epicresearch.org/readmission-ai-study
Source for data showing AI’s role in cutting readmission rates via real-time risk scoring and care coordination.
World Economic Forum (WEF). (2022). Unlocking AI in Healthcare: Telemedicine, Data Analytics, and More. https://www.weforum.org/healthcare-ai-insights
Provided statistics on global telehealth adoption and AI’s potential to fill workforce gaps in mid-sized settings.
Statista. (2023). Healthcare AI Market Size Worldwide. https://www.statista.com/statistics/healthcare-ai-market-size/
Referenced for market growth figures, projecting a surge in AI adoption within healthcare by 2030.
OptumIQ. (2021). Survey on AI-Driven Diagnostics in US Hospitals. https://www.optum.com/resources/reports/ai-diagnostics-survey.html
Cited for examples of how AI-assisted imaging improved detection rates of critical conditions.
Johns Hopkins Medicine Case Study. (2022). Implementing AI-Powered Clinical Decision Support in a Mid-Sized Hospital Network.
https://www.hopkinsmedicine.org/ai-clinical-decision-mid-sized-case
Comentários